Online Payments Fraud Detection with Machine Learning Algorithms
Table of Contents
Table of Contents
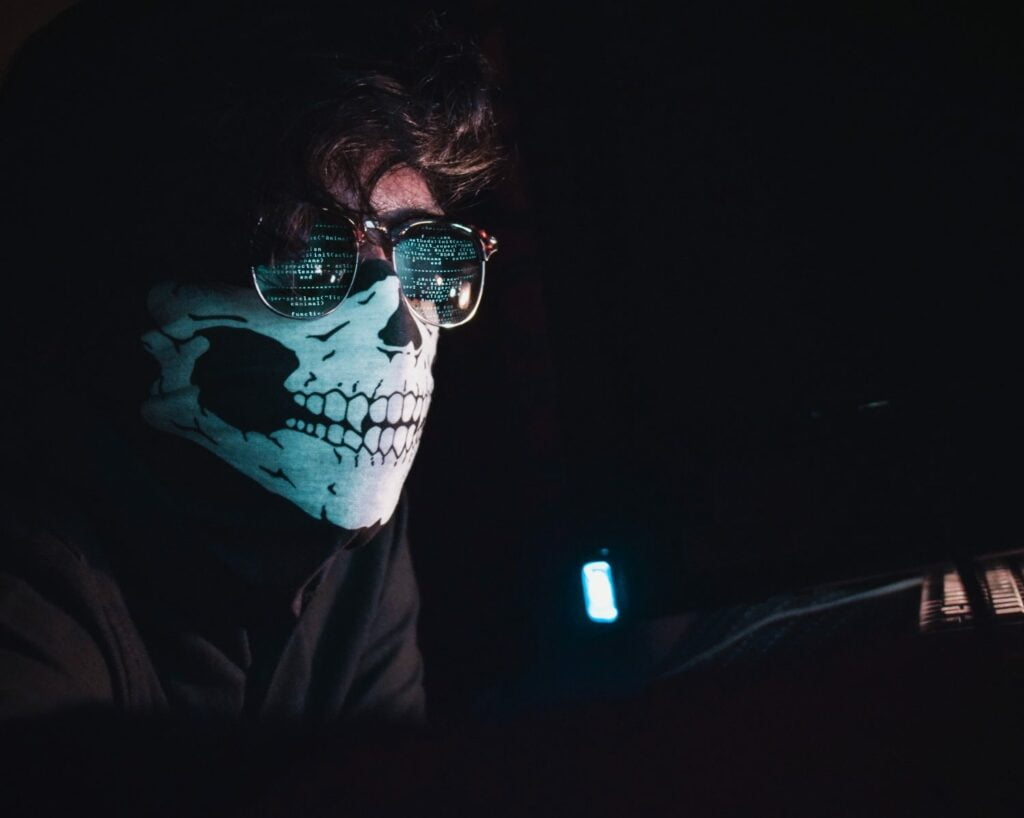
Not only banks and financial institutions use fraud prevention systems. Websites and e-commerce platforms working with customers and dealing with sensitive user data should also use reliable fraud prevention software to detect and prevent attacks and implement fraud prevention methods to life. This article discusses online fraud prevention goals, strategies, and machine learning (ML) fraud detection software to avoid losses caused by fraudulent behaviors.
What is fraud detection?
According to the definition provided by the Cambridge Dictionary, fraud is the “crime of getting money by deceiving people.” Fraud has existed in one form or another for many centuries. Ever since people started to exchange goods and services, there has been a risk of one party scamming another and third parties scamming both sellers and buyers.
Fraud undergoes regular changes and transformations. It’s gained new forms with the e-commerce expansion. Fraudsters take full advantage of every weak spot detected in online banking, online insurance, e-shopping, and other niches. Fraud has become a major issue for e-commerce and banking retailers globally.
Detecting, preventing, and fighting fraud are among the primary concerns in the modern-day world. One of the most effective ways of managing attacks and risks is by using machine learning algorithms for fraud detection.
Machine learning is useful in so many ways. It’s been widely used for email spam detection, providing customer-focused recommendations on e-commerce platforms, and much more. The possibility of working with large data volumes makes machine learning one of the best choices for building fraud detection technologies. Thanks to the constant advancements in machine learning, eCommerce and online banking sectors can take significant advantages in preventing cyber-crime and keeping sensitive data safe.
Types of Fraud Faced by Online Retailers
When it comes to eCommerce fraud, cybercriminals are known for their creativity. Although stealing bank account information is generally considered one of the most common types of fraud faced by online retailers, there are many other ways to target users’ devices and sensitive information. Let’s consider the most widespread types of fraud eCommerce businesses face.
- Payment fraud constitutes a significant 71% of all e-commerce attacks. Cybercriminals often use stolen card details, personal information, and IP addresses to appear as legitimate customers. Payment fraud is commonly used to create fake accounts and purchases, and manipulation of website trafficTrafficThe number of visitors or users who visit a particular website.
More About Traffic. - Friendly fraud can happen intentionally or accidentally. In friendly fraud, a customer claims that a purchased order was never delivered or arrived damaged, making the online retail store resend another item or issue a refund.
- Clean fraud involves fraudulent transactions that appear legitimate, often bypassing fraud detection systems. Using such tactics as tricking account holders or purchasing on fake websites, fraudsters use stolen credit card information to impersonate the cardholder.
- Affiliate fraud happens when malicious actors deceive online merchants into thinking they have customer attention by manipulating traffic. This type of fraud includes such techniques as sending spam emails and using spam emails or popups to create a false sense of user engagement. Affiliate fraud can undermine affiliate marketing programs where businesses generate a commission by sharing links or content.
- Triangulation fraud derives from the three-step process, enticing buyers, stealing their details, and using them for fraudulent schemes. When this type of fraud occurs, scammers create replica websites and lure customers with cheap goods. Such websites may appear in ads and phishing emails.
eCommerce Fraud Prevention Best Practices
Here are some effective practices to prevent and detect eCommerce fraud.
- Address Verification System (AVS). Use an AVS to verify the customer’s billing address by comparing it to the address on file with the credit card issuer. If there’s a mismatch, decline the transaction to prevent the use of stolen credit card information.
- Two-Factor Authentication (2FA). Implement 2FA, requiring users to provide two forms of identification, such as a password and a code sent to their phone. This adds an extra layer of security to protect customer accounts.>
- Fraud Detection System. Utilize a fraud detection system that analyzes customer behavior to identify patterns indicative of fraudulent activity. Machine learning can enhance its accuracy over time.
- Manual Transaction Monitoring. Regularly monitor transactions for suspicious activity, such as large purchases, multiple purchases from the same IP addressIP AddressInternet Protocol address is a numerical label assigned to each device connected to a computer network that uses the Internet Protocol for communication.
More About IP Address, or inconsistent billing and shipping addresses. Investigate further or contact the customer for verification when suspicious activity is detected. - Verified Payment Gateways. Opt for verified payment gateways that offer secure credit card transaction processing. These gateways often have advanced fraud detection systems to reduce the risk of chargebacks.
- Employee Training. Train your employees on fraud prevention, including recognizing suspicious behavior, verifying customer information, and handling chargebacks. They serve as the frontline defense against eCommerce fraud.
- Stay Informed. Keep up-to-date with the latest threats and vulnerabilities in eCommerce fraud. Attend industry events, follow fraud-related news, and regularly review and update your fraud prevention measures.
- Strong Password Requirements. Customers must create strong passwords that combine letters, numbers, and symbols. Enforce password expiration and account lockouts after a certain number of failed login attempts to deter fraudsters.
- SSLSSLSecure Sockets Layer is a cryptographic protocol that ensures secure communication between a client and a server.
More About SSL Encryption. Secure your website with SSL encryption (HTTPS) to protect sensitive customer data, such as credit card numbers and login credentials, from interception by fraudsters. - Set Purchase Limits. Establish purchase limits to prevent fraudsters from making large purchases using stolen credit card information. Limit the amount a customer can purchase in a single transaction or within a specific time.
- Verify Shipping Addresses. Verify shipping addresses by requiring customers to provide contact information associated with the shipping address. Contact customers to verify orders before shipping to prevent fraudulent orders from being shipped elsewhere.
- Maintain Transaction Records. Keep detailed transaction records containing customer information, including names, email addresses, IP addresses, shipping and billing addresses, purchase history, and payment details. Analyze this data to identify patterns and detect suspicious activity.
- Fraud Protection Services. Consider utilizing third-party fraud protection services that employ advanced algorithms, machine learning, and human analysts to identify and prevent eCommerce fraud. Examples include Kount, Signifyd, and ClearSale.
- Geolocation. Use geolocation to compare the customer’s IP address with shipping and billing addresses. If the IP address doesn’t match, it may indicate fraudulent activity, warranting further review.
What is an anti-fraud system?
It’s a set of measures to detect and evaluate online transactions for possible fraud. Anti-fraud systems focus on specific criteria in every transaction to identify suspicious activities. If the transaction doesn’t meet the specified requirements, it scans the transaction one more time and signals derivations from the norm.
Banks and other financial organizations have been actively cooperating to create new fraud detection guidelines in recent years. The self-learning fraud detection system analyzes the data learned from other financial institutions and makes decisions based on their findings. Studies reveal that the number of suspicious bank loans dropped by 15% shortly after implementing anti-fraud systems at banks.
Why use machine learning (ML) algorithms for fraud detection?
Machine learning (ML) is the science of creating and applying algorithms capable of analyzing and learning from past events. ML is the perfect use case in fraud detection and prevention. Machine learning is capable of detecting fraud from legitimate actions.
The idea behind machine learning usage for fraud detection is that fraudulent transactions include elements that legitimate ones lack. Unlike humans, ML can fight big volumes of fraud way faster and in a timely manner. Machine learning can process a raft of information faster and better than most experienced analysts would do. ML algorithms can spot the cases of fraudulent and unhealthy behavior patterns 24/7/365, remember them forever, and prevent further use cases.
Unlike traditional fraud detection models based on static rule-based systems that have proven effective for a long time, fraud detection using machine learning can go even further and solve the issues that the traditional method cannot spot or fight. The weakest point of traditional fraud detection systems is that they heavily depend on human labor. The work of the top analysts is time and money-consuming. Besides, even the top experts may lack the skills, knowledge, and experience of fraud detection algorithms that use machine learning methods.
ML fraud detection can process new data autonomously 24/7 and update its models and patterns immediately. As the data assets of modern businesses become more overwhelming, it’s getting clear that only machine learning can cope with huge volumes of data. ML algorithms can process and learn more information. Thus, higher workloads result in better fraud detection algorithms.
How does ML fraud detection work?
- Before a machine learning fraud detection model identifies fraud, it needs to gather, analyze, segment, and extract the required data pieces. Humans need more time to comprehend massive amounts of information. The more data should be analyzed for machine learning, the better it is for the result.
- Feature extraction is the next step. At this point, the features describing the customer’s location, identity, orders, network, and chosen payment method are added. Lists of investigated features can differ based on the complexity of fraud detection systems.
- Next, ML model receives training sets that teach it to identify and predict fraud. The more information a business can provide for a training set, the better the ML model will be built.
- As a result of these activities create fraud detection ML models suitable for specific businesses as a result of these activities. Such online fraud prevention systems can identify, predict, and fight malicious activities with higher accuracy. When using the ML model for online payment fraud prevention, it’s important to update and improve it to detect new tricks that fraudsters invent.
Machine learning algorithms for fraud detection
- Supervised learning algorithms are used for fraud detection in deep learning environments in FinTech. It’s based on predictive data analysis. Such ML models label all information either as good or bad. The major drawback of this ML model is that it cannot detect fraud that was not included in the historical data set from which it learned.
- Unsupervised learning model is meant for fraud detection in cases where there is little transaction data, or such data is not available. It analyzes patterns and decides whether they are legitimate or fraudulent.
- Semi-supervised learning is somewhere in between supervised and unsupervised learning. This ML model is used in scenarios when labeling information is either impossible or too expensive and requires the labor of human experts. The semi-supervised algorithm stores information about important group parameters even when group membership of the unlabeled data is unknown.
- Reinforcement learning algorithm learns from the environment to detect ideal behavior within a specified context.
ML fraud prevention use cases
Many organizations can keep their finances and sensitive information safe by using machine learning for fraud prevention. The world has already seen examples of fraud detection algorithms used for e-commerce, banking, healthcare, online gaming, and other industries. ML fraud prevention models can be applied to many different businesses regardless of the sectors to which they belong.
The most popular companies whose online fraud detection systems are known worldwide include Capgemini (claims their ML fraud detection system can reduce fraud investigation time by 70% with the accuracy increased by 90%). Feedzai is another example in which machine learning solutions can identify and prevent 95% of all fraud.
Some of the most vivid examples of companies that already use ML fraud detection models include Airbnb, Yelp, Jet.com, etc. Such companies use AI solutions and ML algorithms to get insights from big data and prevent issues such as fake accounts, account takeover, payment fraud, and promotion abuse.
Bottom line
We live at such an amazing time of progress and innovations! The opportunities for business development are limitless. However, we shouldn’t forget about another side of the coin – online fraud – and find reliable methods and algorithms to fight it effectively. Machine learning is the most innovative and dependable fraud prevention tool of the current times. By implementing ML fraud prevention models, businesses become more resistant to hacks and malware of any nature. Relying only on human experts is not enough if an organization wants to make it impossible for trickers to access sensitive information.